Agentic AI is here to stay and is innovating how businesses function. It’s way past being a hyped-up new term on the block. Agentic AI is the present and the future of digital-first companies. The next-gen AI wave has already begun, with the most prominent brands shifting to no-code or low-code platforms and unveiling their own AI agents. In just two weeks, we heard news of Adobe launching the Adobe Experience Platform Agent Orchestrator, Deloitte unveiling Zora AI, Lenovo announcing Lenovo Hybrid AI Advantage with NVIDIA solutions, and Zendesk’s AI-powered platform for customer services.
All of this in just two weeks. That’s how fast Agentic AI is penetrating modern businesses.
The focus has shifted to achieving business KPIs faster with AI agents acting as co-pilots. These AI agents are competent and self-learning, and can think, decide, and execute like humans (but much faster). This is why businesses are ditching standalone large language models for a more transformative AI – Agentic AI.
What is Agentic AI?
Predictive analytics, large language models, RAG systems, and process automation tools have enabled businesses until now, but these technologies still require human input and monitoring. Agentic AI breaks this constraint. It is a self-directed decision-making system. You can say it like – If artificial intelligence is electricity, agentic AI is the bulb.
This transition in AI is already in motion. Businesses that want to leverage AI’s potential must understand and follow this transition.
Agentic AI is a breakthrough capability that will soon lead to fierce competition. The market for agentic AI is in its nascent stage, offering businesses ample scope to understand, learn, and iterate so that these robust systems stay aligned.
Just as they say, with great power comes great responsibility.
Agentic AI is extremely powerful. If companies don’t understand the ethics of AI and the boundaries of end-users when experimenting and iterating with this system, the consequences can do more harm than good.
Agentic AI – Key Features
Agentic AI is an AI system that can act autonomously. These systems can solve multi-step problems based on context and objectives and adapt in real time. Agentic AI tools or systems are built of multiple AI agents that use large language models (LLMs). This helps AI agents understand natural language and enhance decision-making.
Traditional AI and generative AI did make progress in handling business challenges, but when matters got more complicated, they failed to deliver end-to-end enterprise solutions. Conventional AI systems are rule-based and pose many restrictions on how far AI systems can adapt, learn, and make decisions.
Agentic AI systems ensure effectiveness and adaptability in a dynamic tech world. The key characteristics of these systems include:
- Autonomy: Can perform tasks independently without constant monitoring or human oversight.
- Goal-driven: Pursue complex objectives by taking up tasks and reasoning on how to achieve them.
- Execute actions: Can not only identify the optimal way to solve the problem but also execute the required actions to achieve the desired outcomes.
- Scalability: Can handle increased workloads. They are designed to expand functionalities to incorporate businesses’ evolving needs.
- Adaptability and self-learning: These systems can adapt to the environment and respond to new data and scenarios. They can evaluate outcomes, learn from them, and adjust strategies independently.
- Integration with enterprise systems: Can effectively integrate with enterprise systems, operating within existing technology ecosystems.
Agentic AI vs. GenAI vs. Traditional AI
Traditional AI is limited to automating repetitive tasks. It relies on specific algorithms and predefined rules and requires human monitoring.
Generative AI does not require human monitoring as it learns from its existing data. It can answer questions, generate texts, and assist with various daily tasks. However, it cannot make decisions or adapt to changing environments.
This is where agentic AI wins. Agentic AI can take initiatives, execute them, and learn from its experiences. It can adjust its actions over time and handle more complex tasks, including problem-solving and decision-making.
Features | Traditional AI | Generative AI | Agentic AI |
---|---|---|---|
Primary Function | Rule-based or predictive tasks | Creates new content (text, images, etc.) | Autonomous decision-making & goal pursuit |
Learning Approach | Supervised/Unsupervised Learning | Deep Learning (LLMs, GANs, Diffusion) | Reinforcement Learning + Generative Models |
Output Type | Classifications, predictions, decisions | Novel content (text, code, media) | Actions, plans, or multi-step solutions |
Adaptability | Limited to trained scenarios | High creativity but static post-training | Dynamic, self-improving, and goal-driven |
Autonomy | Low (follows predefined rules) | Medium (generates but lacks agency) | High (autonomously acts on objectives) |
Examples | Spam filters, recommendation systems | ChatGPT, DALL-E, MidJourney | AI agents (e.g., AutoGPT, Devin AI) |
Decision-Making | Deterministic or probabilistic | Probabilistic (no “understanding”) | Goal-oriented with reasoning capabilities |
Interaction with the World | None (passive analysis) | None (content generation only) | Yes (can execute tasks in digital/physical realms) |
Key Tech | Decision trees, SVM, regression | Transformers, GANs, VAEs | LLMs + RL, Agent frameworks (e.g., LangChain) |
In a nutshell, traditional AI focuses on analysis/pattern recognition, generative AI focuses on creation, and agentic AI focuses on autonomous actions. Let’s dive into how agentic AI is transforming modern businesses.
Agentic AI and Business Workflows
When agentic AI meets business workflows, we get agentic workflows—a term that resonates with how modern businesses want to function. Agentic workflows are autonomous tasks that are performed automatically without any human monitoring. Imagine recurring tasks like resetting passwords or approving time-off requests being on copilot while you focus on essential strategic tasks. That is what agentic workflows do for you.
Agentic AI is the engine, while an agentic workflow is a structured series of actions that the system performs to achieve specific business goals/outcomes. Automation handles simple repetitive tasks. Agentic workflows take it one step further by analysing and interpreting data and streamlining complex and large-scale processes. Once such a workflow is set up, you and your team are no longer required to fill in gaps, unlike with traditional AI.
If you look at the bigger picture, agentic workflows are set to have a huge impact on business operations. Experts predict that at least 50% of businesses will implement AI pilots by 2027 to handle business operations.
It is not just a hunch; even investors have started realizing the huge potential of AI agents. This has already led to over $2 billion in investments in agentic AI startups.
We are looking at businesses that will use agentic AI to optimize faster, improve processes, scale smartly, and reduce manual errors.
How Does Agentic AI Streamline Processes And Enhance Productivity?
The impact of agentic AI on business operations is directly related to the business process layer in a new-age business architecture. Businesses have interconnected layers, each with distinct responsibilities. Agentic AI agents help transform these business layers and scale it to an unprecedented level, driving automation, adaptability, and intelligence.
A new-age business architecture is multi-layered. Each layer contributes to the smooth running of operations and efficient decision-making. In hindsight, a modern business architecture will have three layers or levels –
- Tech layer – home for the tech platform, and infrastructure components
- Information layer – houses application systems and data components
- Business layer – defines business values, competencies, processes, and service aspects
If you stack these layers and create a visual of the architecture, the layers are arranged as follows:
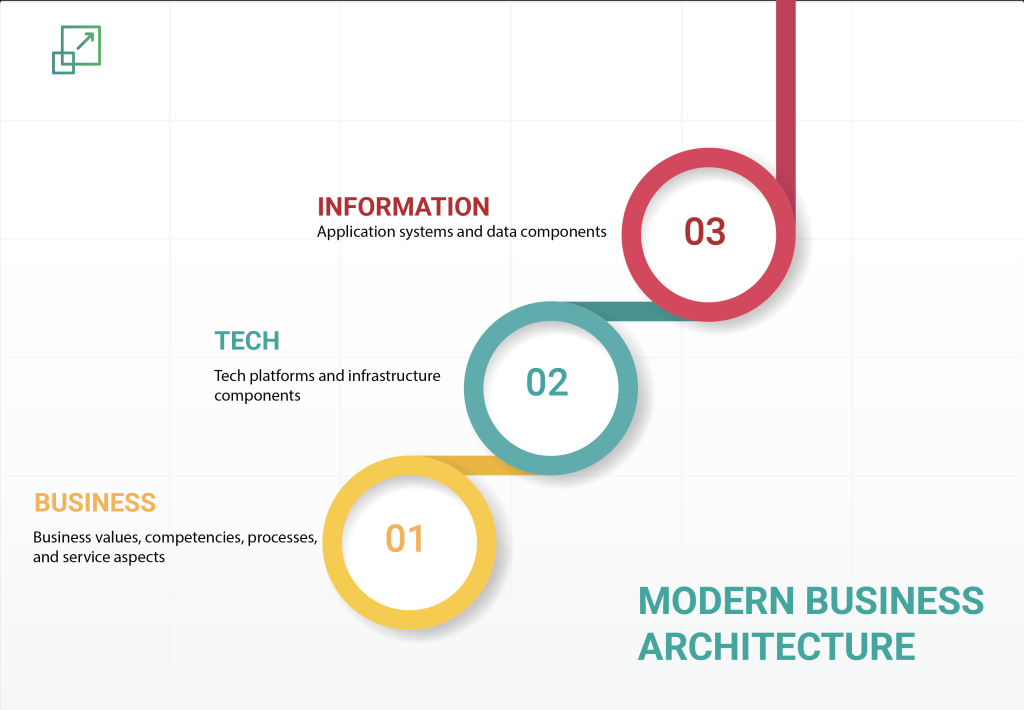
The tech layer is at the bottom, followed by information, and finally, the business layer is on top.Looking at the image above, the business process layer is the bridge connecting enterprise applications and customer interactions. It is in this layer that AI agents find their primary roles.
- Why the business layer?
For most enterprises, agentic AI agents are implemented in the business layer. This is central to streamlining processes and optimizing overall business efficiency. The tech and information layers also require AI agents, but those are very task—and layer-oriented.
The business layer is responsible for structuring and managing business workflows across an enterprise. Everything happens here, from orchestrating processes to workflows, decision logic executions, data coordination, and operational monitoring.
However, business processes still require manual oversight, slowing down execution. Simultaneously, this layer cannot adapt with the dynamic envrironments, often work in silos across departments, and store information separately making it challenging to collate evertyhing and take informed business decisions.
Agentic AI agents negate all these challenges, promoting adaptability, reducing human intervention, optimizing operational efficiency, and scaling intelligence through seamless coordination between different business functions.
These agents can adapt to dynamic environments, analyze vast data and make autonomous decisions, streamline interactions across multiple teams and departments, and handle unexpected scenarios by learning from new data and modifying responses.
AI Agents are Reshaping Business Operations
Agentic AI functions in four levels –
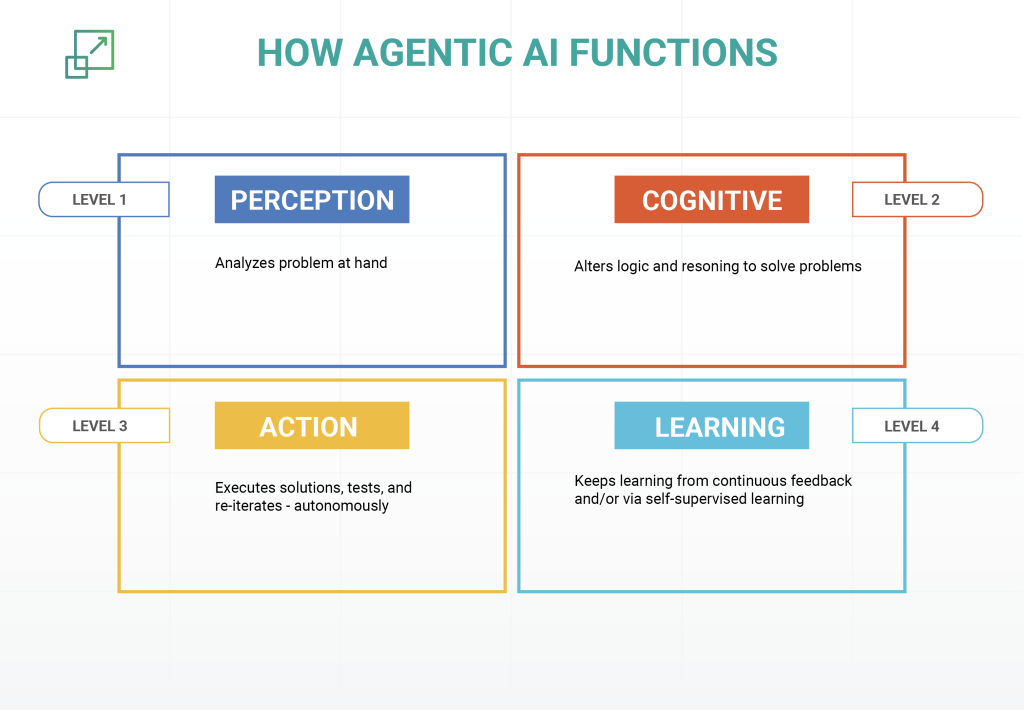
- Perception: where it analyzes the problem at hand.
- Cognitive: where it alters logic and reasoning to solve the problem.
- Action: where it executes solutions, tests, and reiterates autonomously.
- Learning: where it keeps learning from continuous feedback, either via human-in-the-loop or self-supervised learning.
When integrated within a business infrastructure, these four layers improve business efficiency, reduce costs, and help effectively align business goals with strategies. Let us see how.
AI agents can seamlessly automate regular, mundane tasks, such as data entry, invoicing, password reset, answering customer queries, capturing initial lead details, appointment scheduling, email management, and more. This gives team members more scope to focus on business strategies.
1. Automate repetitive tasks
These virtual assistants integrate with business applications to automate cross-platform tasks. For instance, an AI agent can extract an invoice from an email, sync it to accounting software like QuickBooks, and set up payment reminders for the finance team—all of this without any human intervention. This saves time, boosts productivity, and cuts down operational costs. Bonus: The chances of errors are slim.
Example: Microsoft Power Automate is a business copilot that lets you automate mundane tasks by defining your requirements in a simple prompt. You can either use one of their set prompts or explain your specific needs to streamline your repetitive tasks. Get, set, and forget—that’s how automation with Copilot works.
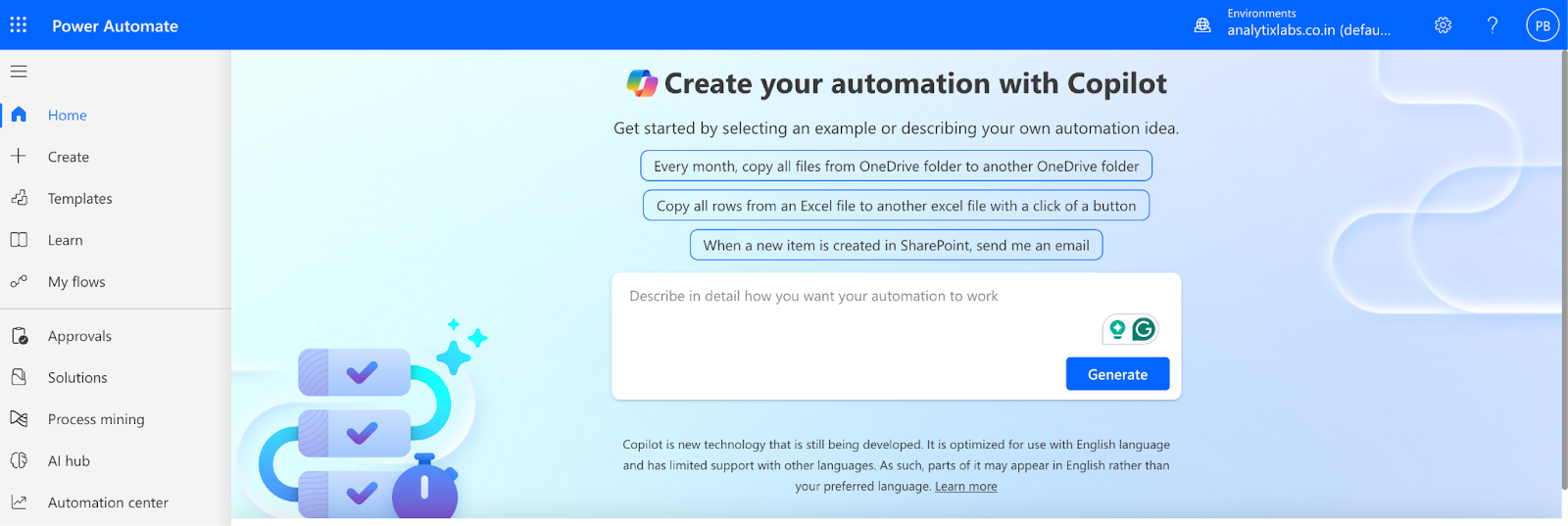
2. Autonomous decision-making in real-time
Imagine opening a travel booking app like MakeMyTrip and seeing different prices for the same flight route on different days, or how fares change when booking a round-trip ticket compared to a one-way ticket. This surge pricing algorithm autonomously adjusts fares based on airport traffic, flight distance, flight frequency, time of flight, dates, demand, and your level of urgency.
Here is an example of flight fare differences between Indian cities, such as Bangalore to Hyderabad, in August. The pricing is higher around August 14 and 15, as Independence Day is a national holiday in India and falls closer to a weekend, which suggests that more people might travel on those days. Hence the surge price.
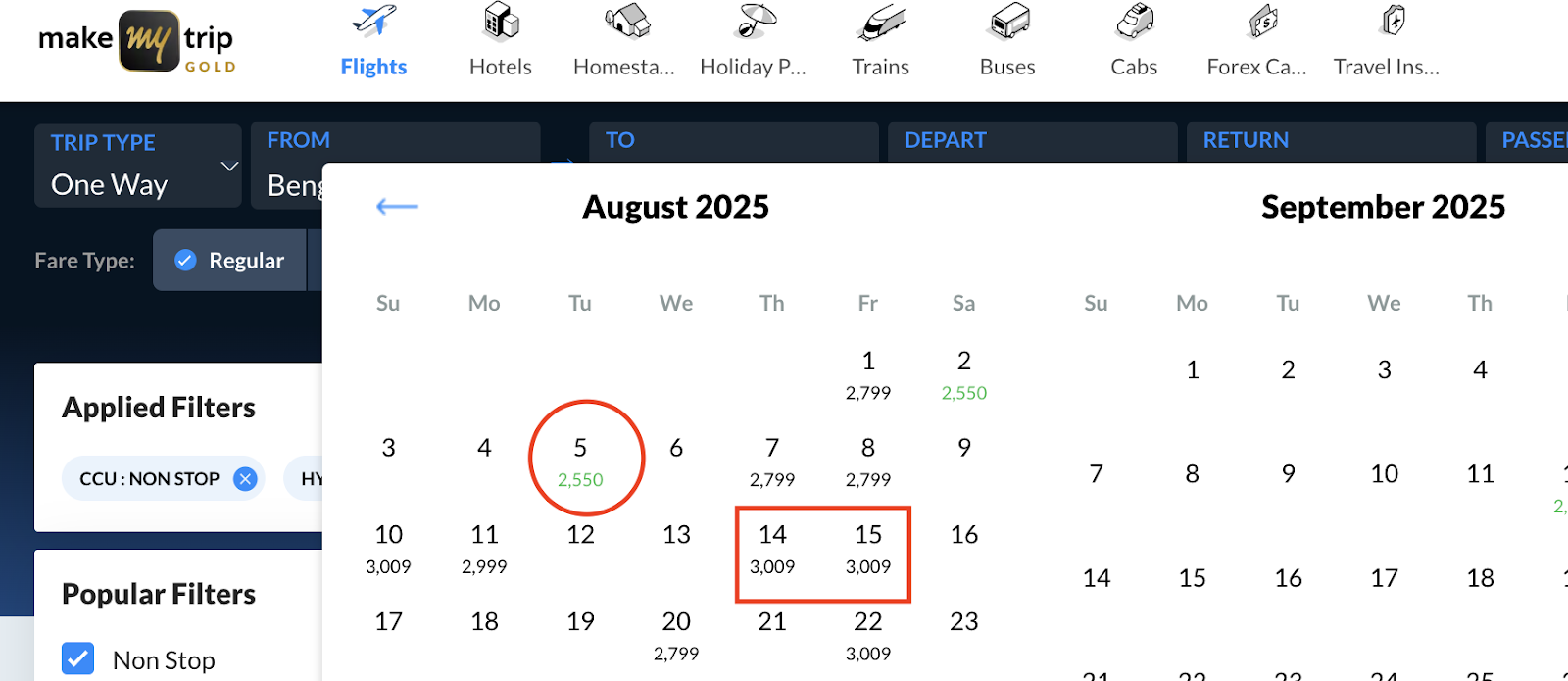
Suppose you are comparing, exiting, and then returning to look at the same route, or browsing hotels for a destination you have already browsed. In that case, the algorithm will capture the urgency affecting the pricing you see. A similar surge is often observed in mobile browsing compared to web browsing within the same app.
These AI agents use reinforcement learning to continuously improve their decision-making, learning from past outcomes and refining future actions.
Another example is Netflix’s AI, which can automatically adjust the streaming quality in real time based on users’ internet speed and device. This helps smooth playback and ensures uninterrupted user engagement.
3. Enable customer service and support
Leveraging agentic AI to engage customers and deliver support on time is crucial for any business. It’s not always about implementing a chatbot that answers initial queries and captures lead information. Imagine having an AI assistant that will tell you how to convince a potential user and convert them into a paying customer? How easy that would be!
Gong.io, an AI platform revolutionizing sales, is doing precisely that. Gong.io helps analyze call recordings, offers suggestions to sales teams on negotiation tactics, and identifies opportunities for upselling.
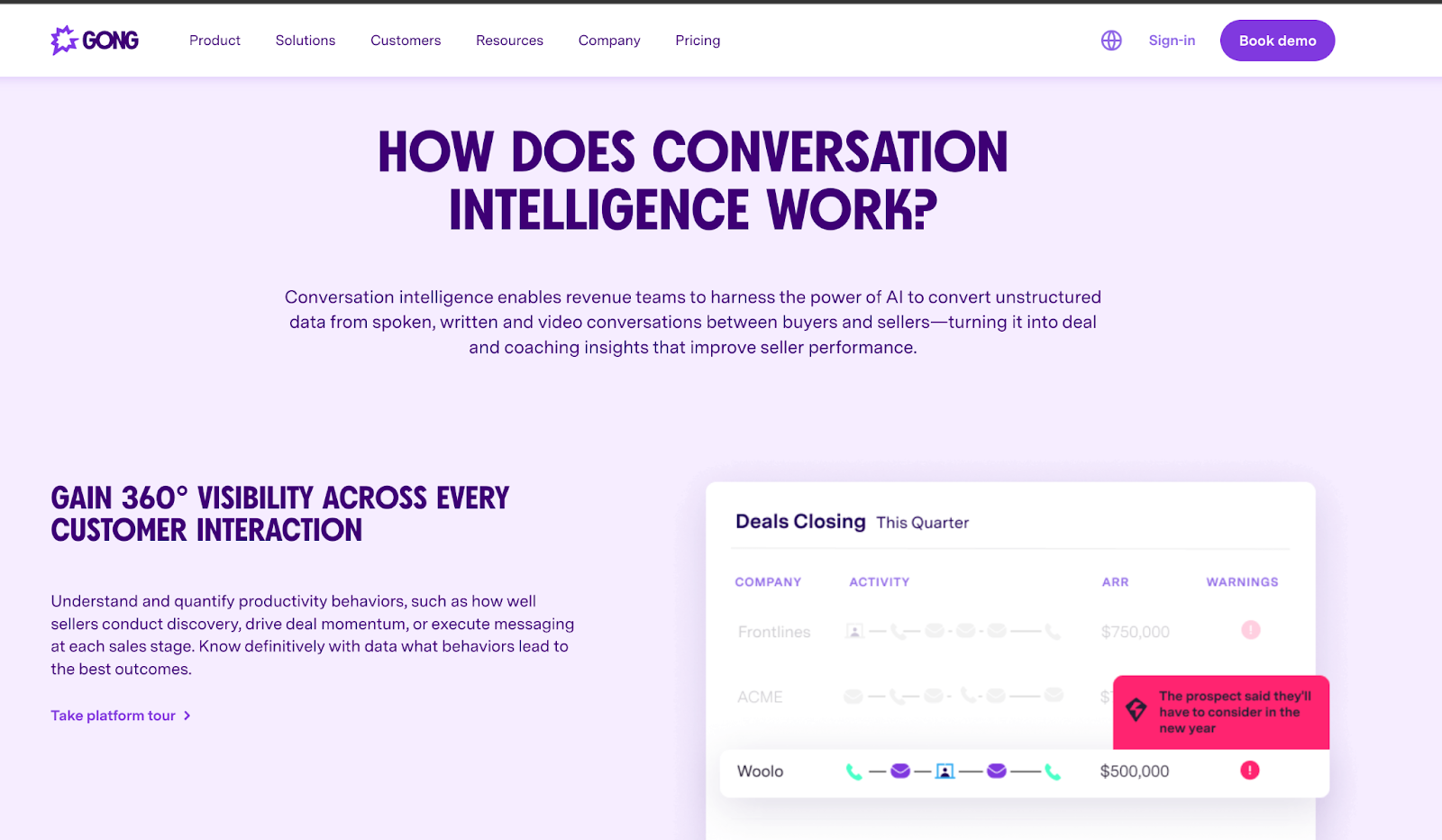
This is the kind of AI assistance businesses are investing in. AI agents that can do more than send automated responses. These AI systems leverage natural language processing (NLP) and sentiment analysis to understand user intent, need, urgency, and potential.
Accordingly, these agents can create tactics that help deliver proper support to the end user and also highlight potential upselling opportunities. AI agents are smartly assisting businesses in generating revenue, not just providing customer support.
Modern AI systems handle complex customer interactions, negotiate deals, and personalize recommendations. For instance, Airbnb’s AI pricing tool suggests optimal rental prices based on factors like local events, season, and competitor rates. This helps hosts maximize bookings.
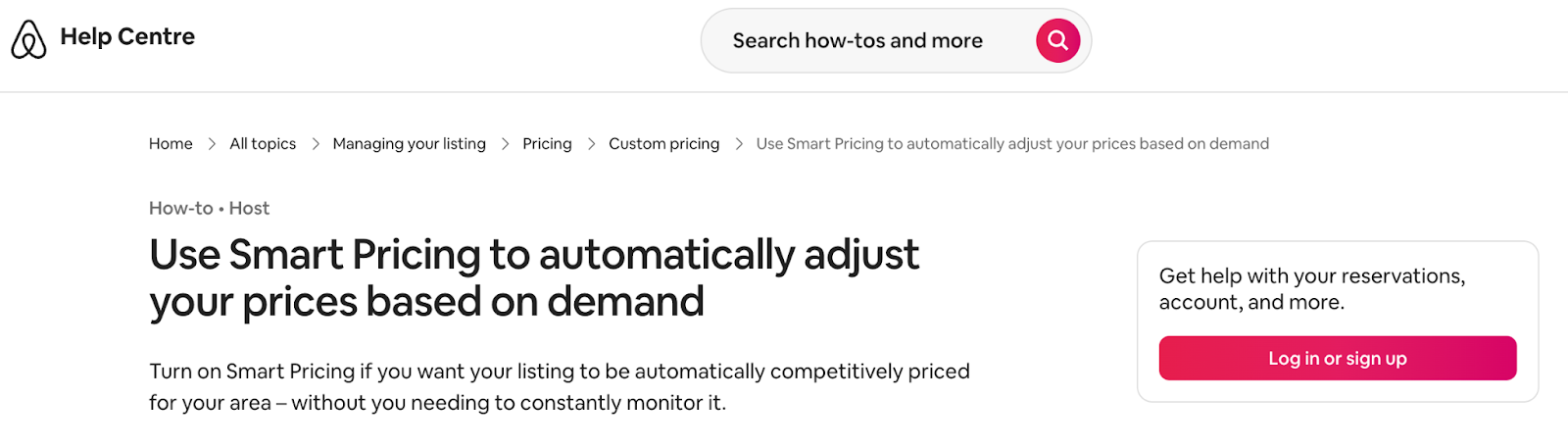
4. Help in fraud detection and safeguard user data
PayPal, a leading name in payment gateway, has access to millions of users’ data, including transaction details, bank details, card details, location, email, computer IP, and more. Imagine a fraud alert for a company like this. The massacre is beyond comprehension.
This is where agentic AI systems are stepping up the game. PayPal’s AI-powered fraud detection system can analyze $1.5+ trillion in annual transactions with 99.9% accuracy. The AI maps transaction patterns such as purchase frequency, device fingerprints, and IP locations to detect anomalies. Simultaneously, it tracks subtle user behaviours like typing speed and mouse movements to identify whether it is a human user or a bot at the other end.
Agentic AI systems can identify fraud faster and automatically block such activities. PayPal’s AI blocks $20+ billion in fraudulent activities, such as credit card theft and account takeovers.
These AI systems reduce false positives. Unlike rule-based systems, agentic AI minimizes legitimate transactions that are mistakenly declined, keeping customer frustrations at bay. They learn continuously and update models based on new fraud tactics, like the very recent generative AI scams.
With such AI systems in place, businesses using tools like PayPal can remain calm while handling hefty business transactions (that often include payments in global currencies).
5. Revolutionize marketing and advertising for enterprises
AI agents can analyze market data and help businesses deliver targeted ad campaigns and marketing content. Brands like Nestlé and Coca-Cola are already creating AI-generated ad campaigns that are hyper-targeted, personalized, and aligned to how their end users connect with the brand.
This is one side of the coin. Marketing ad budgets are often dynamically adjusted by AI agents to fit market demands.
For instance, the Atlanta beverage giant Coca-Cola’s AI analyzes real-time sales data, weather trends, and social media sentiment to adjust ad bids automatically. The AI will increase the bids for cold drink ads in regions affected by heat waves at a specific time of the year. It also shifts budgets between platforms like Google, TikTok, and television based on predicted ROI (using reinforcement learning).
This autonomous decision-making has seen a 20% boost in Coke’s sales. Timely and context-aware campaigns, like broadcasting Coke’s campaigns during a sports event, have helped the brand leverage AI to churn maximum ROI.
AI agents are changing how brands market.
What was once a 100% tried-and-tested method has now evolved to dynamic marketing, constantly shifting and adjusting with trending user demands in real time. Humanly, this kind of dynamic shift is too much to implement, but for AI bots, it happens in a snap.
Marketing is not only about ads. Many layers fall under marketing. One is engaging users. AI systems can enhance how you interact and engage your users on your platform.
For instance, global music streaming app Spotify uses AI to help write playlist descriptions. The AI can analyze song lyrics, tempo, and the listener’s mood to write descriptions of the playlist.
If a playlist has high-beat songs or is a workout playlist, it will analyze the lyrics and tempo, and automatically create a description like high-energy music to help you crush your day. It does the same with podcasts streaming on the app.
From creating mood-based playlists to reiterating descriptions with different phrases to maximize saves, Spotify’s AI algorithm is making every playlist feel personal.
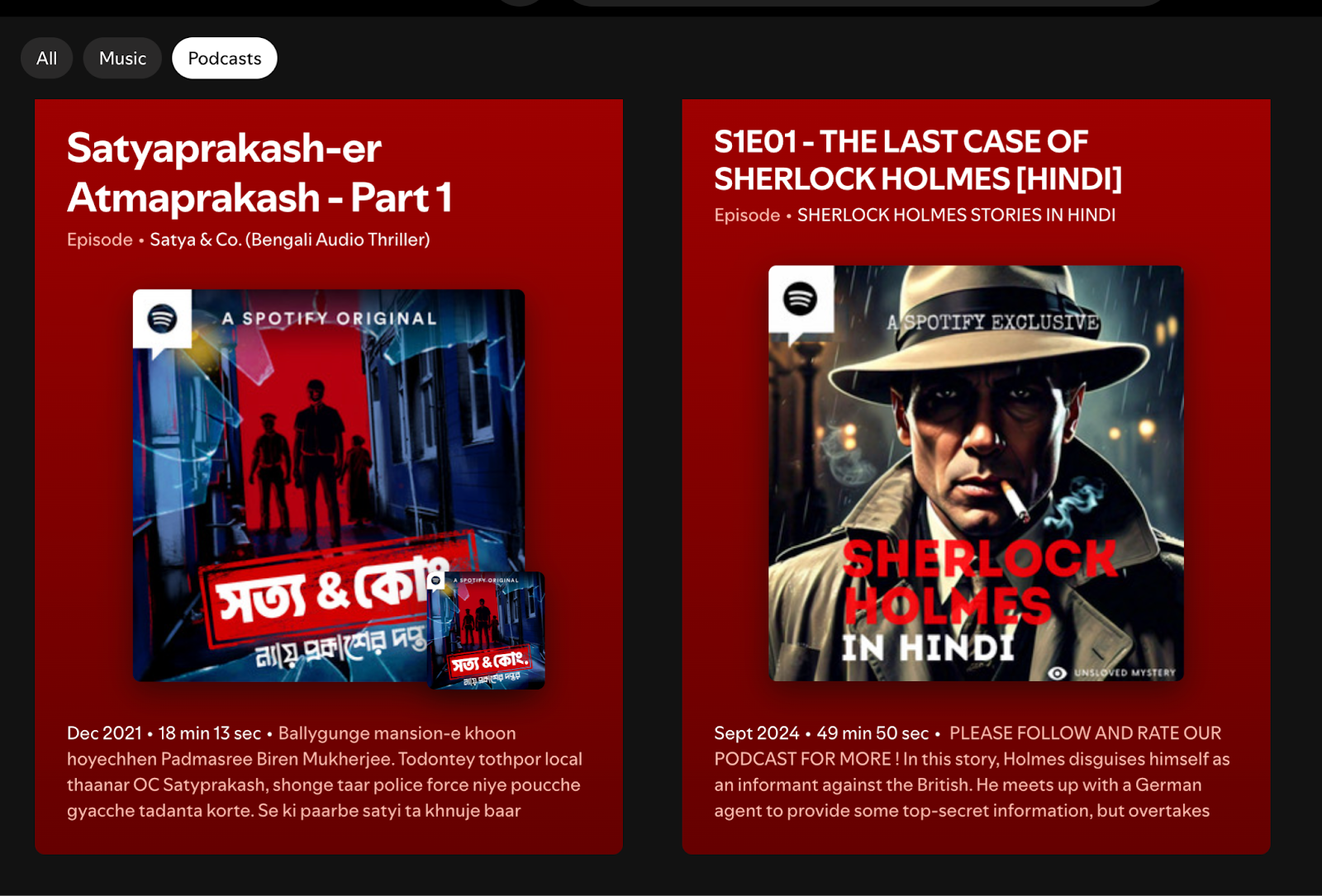
Benefits of Implementing Agentic AI in Organizations
You know about agentic AI’s adaptability, scalability, and autonomous decision-making features. In addition to these, agentic AI has other aspects that benefit businesses of all sizes.
- Scalability
Agentic AI solutions are designed to scale with businesses seamlessly. They can manage high workloads without increasing resources. For instance, during peak seasons or limited-sale offers, eCommerce giants like Amazon often rely on AI to handle heavy traffic without compromising on quality and delivery promises.
- Fosters collaboration
Agentic AI can foster a productive environment by taking up repetitive tasks that cause employee burnout. This gives employees time to focus on their core responsibilities and contribute effectively to business goals.
For instance, Hubspot’s marketing teams use AI to automate data analysis and reporting tasks while the team focuses on strategy development. Similarly, tools like Slack leverage AI to keep teams connected, automate workflows, and suggest actions.
- Streamlined compliance
Using AI is fun and interesting, but only when it adheres to compliance boundaries. Adhering to regulatory requirements is resource-intensive and complex for many organizations, especially those in heavily regulated industries like healthcare and finance.
Agentic AI systems can automate compliance monitoring and reporting, making it easy for organizations to function within set boundaries. For instance, financial institutions and banks can leverage AI to detect suspicious transactions, ensuring complete compliance with anti-money laundering regulations with minimal manual oversight.
- Inventory and supply management
Overstocking and understocking can disrupt business and lead to losses for e-commerce and retail companies. Agentic AI algorithms can analyze and predict market demands and automatically help optimize inventory. This ensures firms meet customer demands on time without exceeding costs in overstocking or understocking.
For instance, a bookstore can use an agentic AI algorithm to analyze end-user responses to newly published books and accordingly refill stocks to meet increasing demand.
- Accelerate time-to-market
Let’s say you are building a product that will solve a pressing social issue and is urgently needed in the market. However, the long product development cycle is delaying your product. Probably, by the time it is ready, some other competitor will take away the market.
Agentic AI algorithms can accelerate product development timelines, giving businesses the edge to compete in the market effectively. They can streamline processes and improve inter-team collaborations, shortening the timeline to develop and launch a product.
For instance, pharmaceutical company Pfizer uses agentic AI in the drug discovery process. AI helps analyze large datasets rapidly, cutting short the development timeline and accelerating treatment for critical diseases.
Challenges with Agentic AI in Business
The possibilities with agentic AI are unprecedented, but businesses continue to face roadblocks when trying to implement agentic AI algorithms into the tech stack.
While the movement towards open-source frameworks and no-code/low-code tools is reducing friction by a fraction, the challenges of technical complexities, high setup costs, and ethical bias remain.
No-code/low-code platforms offer a plug-and-play solution to various regular business tasks. However, the real challenge arises when an enterprise wants to integrate an agentic AI algorithm at the center of its tech stack.
Research shows that security concerns are one of the top reasons for not deploying AI systems immediately, closely followed by the complexity of development, integration challenges, and the lack of skilled professionals who can handle such complex frameworks.
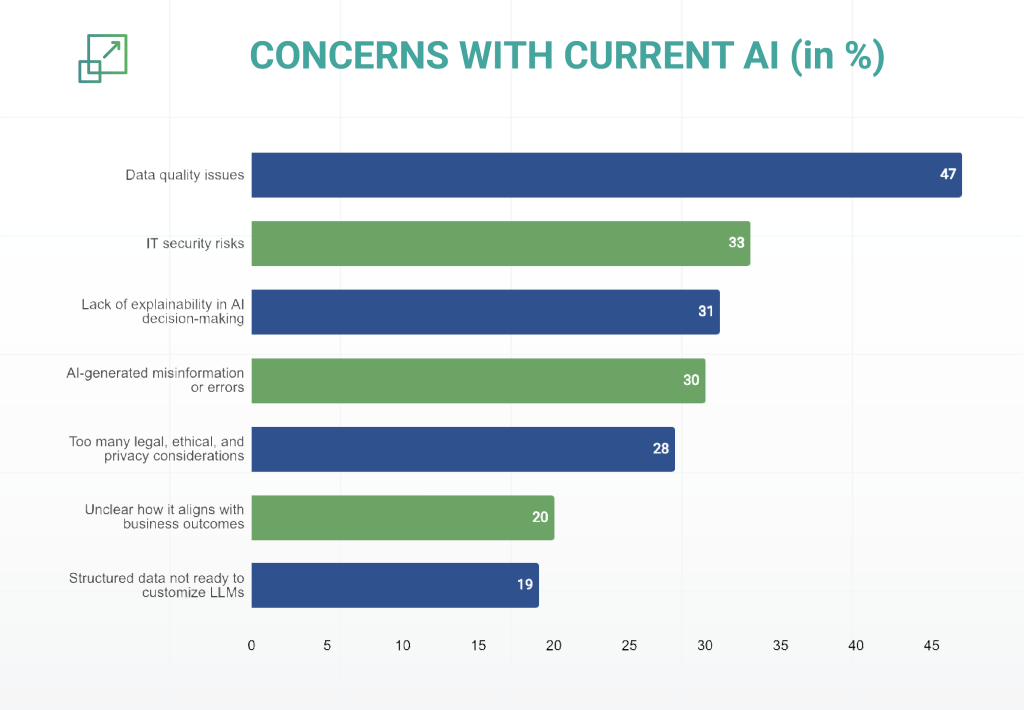
The challenges are not limited to security or a lack of skilled professionals. With generative AI and LLMs gaining momentum, new concerns are emerging around data quality, the explainability of results, and transparency on how AI is aligning with business goals. Below are the top challenges enterprises face with agentic AI.
- Unforeseen consequences
Agentic AI can make decisions autonomously, which is great. However, this can lead to unforeseen actions or decisions, leading to consequences for which businesses are seldom prepared. While these algorithms can self-learn and adapt, there is a chance that they perceive surrounding information incorrectly, leading to the wrong set of actions.
- Limited understanding of how it works
The tech part is highly complex, and so is the decision-making process. Incase there is an error or misjudgment, it is difficult for businesses to track down the root cause of the error. This makes AI algorithms opaque, posing a threat to businesses time and again. Most agentic AI models, especially the deep learning systems, operate as ‘black boxes’ – businesses cannot fully understand how they make decisions.
- Lack of transparency in data usage
Agentic AI algorithms can analyze large datasets at lightning speed. This also leaves room for misuse of user data. Businesses are still concerned about the potential misuse of user data, which could put them in peril should there be any consequences of the misuse. Businesses require transparent practices in data collection, storage, and utilization.
- Ethical and bias risks in decision-making
Agentic AI systems learn from historical data. However, this data can contain societal biases, discrimination, or flawed business practices. AI models lack human moral reasoning, raising concerns for businesses since not every business has access to tools that can detect hidden biases.
- Security vulnerabilities and AI manipulation
Agentic AI models are statistical systems (not rule-based). Adversarial attacks subtly alter inputs, like adding pixel-level noise to an image, that can fool facial recognition AI into misclassifying identities.
So, when businesses prioritize functionalities over security, they leave gaps for exploitation.
Human-AI Collaboration: Need of The Hour
Despite the concerns around bias and security, there is no denying that agentic AI is reshaping how businesses function. From recruitment to product development, from coding to creating business presentations, and more – agentic AI is enhancing how employees function within an organization to achieve set goals.
Is agentic AI replacing jobs?
As more businesses start adopting agentic AI solutions, the need for human labor for repetitive tasks will die out. However, the truth is, agentic AI is changing job responsibilities and making them more integrated into business strategies. Hiring one person for data entry is a waste of resources, but hiring an Excel expert who can use AI to clean data, create reports, generate new formulas, and integrate other tools with Excel to enhance data handling is possible. So the catch here is – upskilling and learning to work with AI assistance.
Businesses need AI experts who know what prompts to use to get work done. Research shows that most businesses show interest in agentic AI because it improves and automates complex business workflows.
The next-gen work culture is all about human-AI collaboration. AI will take the burden of automating regular tasks while you focus on strategies and leveraging AI to deliver requisite outcomes.
How? By understanding how to integrate agentic AI in workflows successfully.
Steps to Integrate Agentic AI in Business Workflows
Your task is to integrate agentic AI in ongoing and new business workflows. The first step is to understand the use cases you are trying to solve and then set clear goals. Remember, these must align with your organization’s readiness to implement agentic AI algorithms.
- You must be clear on what success means for your business. It may differ from business to business. So, make sure you are clear on what your organization wants to achieve with agentic AI—is it customer service or automating operations? Pinpoint the specific operational challenges that are a roadblock for your teams and focus on repetitive and error-prone tasks.
- Know if your organization is ready for agentic AI algorithms. Get a complete understanding of your organization’s technical infrastructure, data quality, and computational resources. Speak to your tech teams to understand their capabilities and expertise and consequently plan for training sessions before deploying AI models. Additionally, speak to your stakeholders to learn about the budget plans to invest in AI tools and training.
- Know what you need. You might need to develop a no-code/low-code tool internally, or you can simply use a plug-and-play tool that offers the solutions you need. This largely depends on what you want to achieve and to what extent. Select tools that align with your needs and goals. Also, it makes sense to look for AI tools that offer APIs and SDKs for faster integration into existing systems.
- Implement AI systems in a phased approach. Begin with low-risk pilot projects, refine your strategy, and slowly scale up. Once you are successful, you can start implementing agentic AI across all business functions.
- Define the points where humans will step in. Agentic workflows will have inflection points that require human oversight so that quality and correctness are not compromised. These checkpoints are crucial to ensuring the workflows continue seamlessly and there are no missteps along the way.
- Set up a feedback loop. Agentic AI improves through a continuous feedback loop. The data from each intersection is used to refine the models and enhance performance. Agentic AI adapts and applies fresh knowledge to help companies become more effective. Set guidelines to segregate feedback that drives value from knowledge-based feedback so that the agents can integrate feedback into the workflows consistently.
Future of Agentic AI in Business: A Kaleidoscopic View
Companies have only just started to realize the potential of agentic AI, yet the growth is massive and market penetration deep.
Microsoft Chief Satya Nadella believes in the impending collapse of SaaS companies in the agentic era. He explained how the core notion of business applications existing will become obsolete because these applications are just CRUD databases with multiple business logics.
Agentic AI is already positioned to dominate these business logics, and it is only a matter of time before these AI agents will become multi-repo CRUD. Nadella believes these agents will not discriminate between what the backend is and will update multiple databases. Thus, all the logic will be in the AI tier.
Resonating with this notion, Satya Nadella also unveiled CoPilot Studio during the Microsoft AI Tour in Bengaluru, India. CoPilot (a no-code tool) will help users create new agents based on their business needs. Nadella said developing AI agents should be as simple as creating an Excel sheet. CoPilot is his UI for AI.
The agentic AI market is evolving at lightning speed. More and more innovations are hitting the headlines, solving business problems – from simple to complex. As the key enabler of no-code/low-code platforms, Agentic AI is helping businesses shift focus to building customized AI agents that will solve core business problems.
Agentic AI is disrupting how common people will access AI with the no-code/low-code tools. It is democratizing technology for a diverse population by simplifying the complex process, empowering non-tech users, and enabling rapid upskilling.
This is the future we are heading into. As traditional roles evolve into AI-driven roles, the world is in a transition phase, steadily adopting the new-age AI, while cautiously treading the boundaries of AI ethics, bias, and user security.
We are not far from the future when these AI-enabled improvements become mainstream across domains and industries. Agentic AI will bring consistency in process, improve efficiency, and reduce operational costs, while adhering to regulatory compliance.
Get ready to see a world full of AI-enabled solutions for what once had no alternative but extensive manual labor, and more.